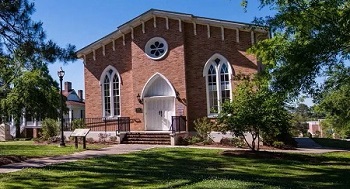
Honors Theses
Date of Award
5-2024
Document Type
Undergraduate Thesis
Degree Name
BS
Department
Chemical and Biomolecular Engineering
Faculty Mentor
Brooks D. Rabideau
Advisor(s)
Kevin N. West, Grayson Dennis
Abstract
Thermally robust ionic liquids hold promise as high temperature solvents, heat transfer fluids, and high-performance lubricants. These ionic liquids, inspired by thermoplastics like PEEK and PES, incorporate peraryl functional groups that lend them high thermal stability, however they often have melting points greater than 100 °C. A broad liquid range is usually desirable in most applications, so finding thermally robust ILs with lower melting points would significantly enhance their applicability. In this study, machine learning models are developed to make predictions of melting points for these thermally stable ionic liquids using a process called quantitative structure-property relationships (QSPR). The methodology used to model these ionic liquids was adapted from a previous study that examined multiple different machine learning techniques in depth. Modeling was performed on the large and diverse set of 929 ionic liquids from this study, then on a set of 53 thermally stable ionic liquids, then on the combined data set. The performance of each model was evaluated using 10-fold cross validation and the calculation of Q2, which measures the predictive capacity of the model. This cross validation only considered the predictions of thermally stable ionic liquids since that is the overall intent of the modelling. From this cross validation, it was found that the model trained on both sets of data and had no outliers removed showed the greatest predictive capability (with a Q2 of .677) for thermally stable ionic liquids. This model is then used to predict ionic liquids from the thermally stable family with low melting points.
Recommended Citation
Morris, Jarod, "Melting Point Predictions of Thermally Stable Ionic Liquids Using a Machine Learning Approach" (2024). Honors Theses. 46.
https://jagworks.southalabama.edu/honors_college_theses/46