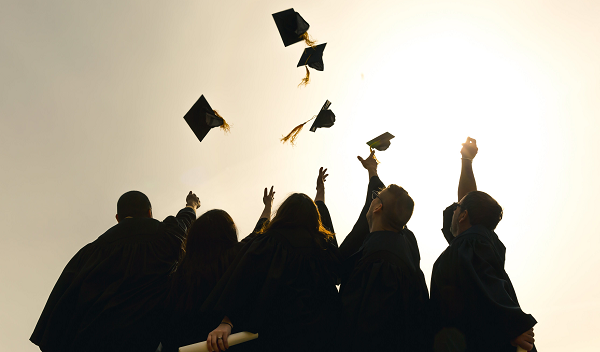
Theses and Dissertations
Date of Award
8-2023
Document Type
Thesis
Degree Name
M.S.
Department
Computer and Information Science
Committee Chair
J. Todd, McDonald, Ph.D.
Abstract
Epilepsy is a recurrence of seizures caused by a disorder of the brain in over 3.4 million people nationwide. Some people are able to predict their seizures based off prodrome, which is an early sign or symptom that usually resembles mood changes or a euphoric feeling even days to an hour before occurrence. Consequently, the natural instincts of the body to react to an upcoming attack lends credence to the existence of a pre-ictal state that precedes seizure episodes. Physicians and researchers have thus sought for an automated approach for predicting or detecting seizures.
In this research, we evaluate the image-based representation of EEG as a basis for classification and training of machine learning algorithms. We explore only the raw EEG data for images in lossless image file formats, though there are other forms including symbolized and noise-filtered that can be explored. Furthermore, we evaluate different color mapping schemes (symbolized, default, chromatic, and binned) that assign EEG data values to Red-Green-Blue (RGB) pixel values. We report the performance of machine learning algorithms such as Random Forest to accurately classify EEG-based images as either event (with a seizure) or non-event (without a seizure).
Recommended Citation
Surles, Amber, "Epileptic Seizure Classification Using Image-Based Data Representation" (2023). Theses and Dissertations . 158.
https://jagworks.southalabama.edu/theses_diss/158
Included in
Artificial Intelligence and Robotics Commons, Investigative Techniques Commons, Medical Neurobiology Commons, Neurology Commons, Neurosciences Commons, Numerical Analysis and Scientific Computing Commons, Other Analytical, Diagnostic and Therapeutic Techniques and Equipment Commons, Other Computer Sciences Commons