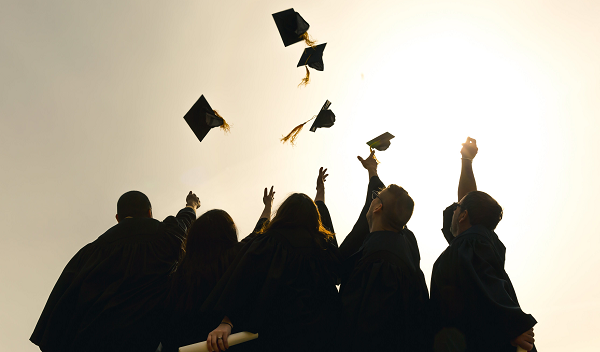
Theses and Dissertations
Date of Award
5-2024
Document Type
Thesis
Degree Name
M.S.
Department
Electrical and Computer Engineering
Committee Chair
Edmund Spencer
Advisor(s)
Purbi Adhya, Mohamed Shaban, Silas Leavesley
Abstract
This thesis aims to develop a hybrid physics-incorporated neural network model (PINN) for classifying geomangnetic substorms in Earth's Magnetosphere. The model is trained using a comprehensive list of substorm onsets, ground magentometer data from a global network, and solar wind parameters from the Advanced Composition Explorer (ACE) satellite. Two different neural network architectures are used, and the physics model used for training is called WINDMI. The magnetic field components on the ground, which are a function of the ionospheric currents, are captured by the SML index. The methodology involves using 60-minute data segments preceding an event to train the hybrid PINN model to predict and classify substorms in the subsequent 30 minutes. A significant part of this research is predicting substorm occurrences based on substorm onsets from four distinct lists and an aggregated substorm list. The results, based on the performance of 16 adopted cases, show that the hybrid model performs significantly better then the WINDMI model alone. This finding underscores the importance of scrutinizing the physics model alongside the hybrid model.
Recommended Citation
Yasmin, Ruthba, "Geomagnetic Substorms Prediction Model Using Combined Physics-Based and Deep Learning Modeling Techniques" (2024). Theses and Dissertations. 193.
https://jagworks.southalabama.edu/theses_diss/193
Included in
Atmospheric Sciences Commons, Engineering Physics Commons, Other Engineering Commons, Other Physical Sciences and Mathematics Commons, Other Physics Commons