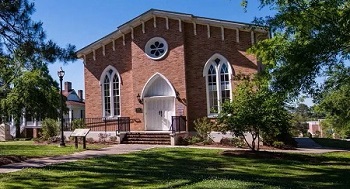
Honors Theses
Date of Award
12-2024
Document Type
Undergraduate Thesis
Degree Name
BS
Department
Electrical and Computer Engineering
Faculty Mentor
Na Gong
Advisor(s)
Mohamed Shaban, Samuel Russ
Abstract
Diabetes Mellitus (DM) refers to a set of metabolic disorders which are generally characterized by an inability to produce or use insulin, a hormone which enables the metabolization of glucose in the blood. Blood glucose (BG) levels are an important health indicator in the management of DM. Conventional methods of measuring BG use direct blood samples and electrochemical analysis. While accurate, these ‘invasive’ methods can cause discomfort, tissue damage, and pose a risk of infection for the patient. Developing a truly ‘noninvasive’ method of measuring or approximating BG has grown increasingly relevant as technology advances and cases of DM continue to rise. In particular, recently, AI-empowered techniques have shown promising results in BG prediction. However, existing techniques are costly and usually need support from the internet, smart phones, or a backend server/cloud. This thesis designs, develops, and prototypes an AI-empowered wearable device (WD) which combines two methodologies for estimating BG and explores the validity of having such estimations performed on a purely edge-based device. The BG estimation methods explored for this device are photoplethysmography (PPG) and breath acetone measurement, with an AI model used to make estimations based on this data.
Recommended Citation
Young, Luke, "AI-Accelerated Smartwatch for Blood Glucose Estimation" (2024). Honors Theses. 90.
https://jagworks.southalabama.edu/honors_college_theses/90
Included in
Biomedical Commons, Electrical and Electronics Commons, Endocrine System Diseases Commons, Endocrinology, Diabetes, and Metabolism Commons, Nutritional and Metabolic Diseases Commons, Other Electrical and Computer Engineering Commons
Comments
© 2024 Luke Young ALL RIGHTS RESERVED