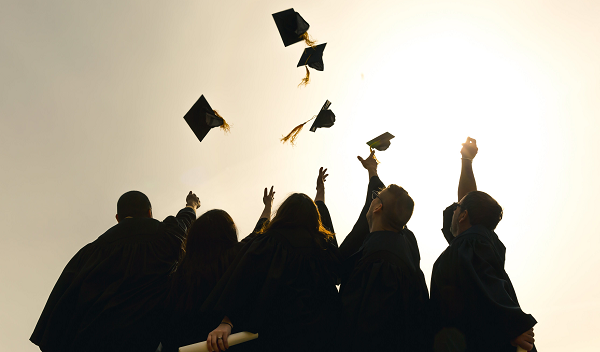
Theses and Dissertations
Mitigating Non-Ideal Characteristics of Memristor-Based DNN Hardware
Date of Award
12-2022
Document Type
Thesis
Degree Name
M.S.
Department
Electrical and Computer Engineering
Committee Chair
Jinhui, Wang, Ph.D.
Advisor(s)
Dr. Mohamed Shaban, Dr. Silas Leavesley
Abstract
Memristor based hardware development has recently received increased attention in academia and industry for edge computing applications, owing to its power efficiency and low latency in performing complex analog in-situ matrix-vector multiplication which is critical to operation in deep neural networks (DNNs). However, the Stuck at Fault (SAF) and nonlinearity behavior of memristors makes such memristor based DNNs inefficient in practice. For SAF, a mapping transformation (MT) method is proposed to increase reliability by mitigating SAF in memristor based DNNs. The weight distribution for the VGG8 model using the CIFAR10 dataset is analyzed and investigated firstly. The MT method is then utilized to obtain inference accuracies ranging from 0.1% to 50% SAFs. The results of the experiments reveal that the MT method can restore memristor based DNNs to their original accuracies. For nonlinearity, the programmed weight for nonlinearity method is proposed to alleviate weight update error in the training process and enhance the inference accuracy even at extreme nonlinear conditions. This method is verified for different technology nodes, device variations, and neural network architectures. Finally, both techniques provide great advantages for improving the overall performance of memristor based DNNs when compared with the state-of-the-art.
Recommended Citation
Khan, Saleh Ahmad, "Mitigating Non-Ideal Characteristics of Memristor-Based DNN Hardware" (2022). Theses and Dissertations. 112.
https://jagworks.southalabama.edu/theses_diss/112