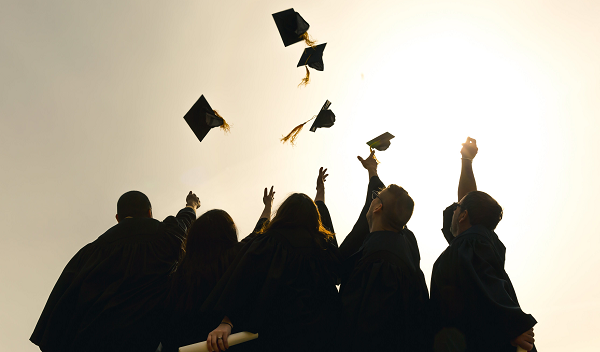
Theses and Dissertations
Date of Award
12-2024
Document Type
Thesis
Department
Computer and Information Science
Committee Chair
George Clark
Advisor(s)
J. Todd McDonald, Arie VandeWaa
Abstract
Computing side-channel research explores the manner in which physical emanations from systems can be used to reconstruct data. Acoustic side-channels are those physical emanations that produce a sonic frequency that is subsonic, supersonic, or considered in the range of human hearing [1]. Acoustic side-channel attacks (SCAs) are typically performed passively: a listening device captures aural frequencies from a machine via a microphone that are transmitted to the attacker for analysis [1]–[3]. Machine learning models have been presented to classify individual keystrokes according to variations in acoustic frequency [4]. Furthermore, the SonarSnoop framework presents a novel active approach that involves both generating and recording aural frequencies acting as a type of sonar system to record physical motion [5].
This research attempts to develop a supervised machine learning model to classify finger motion to collect login credentials typed on a laptop keyboard. The active acoustic side-channel has been used to track two-dimensional finger motion, but three-dimensional finger tracking using active acoustics is novel. The model as trained in this study incorrectly inferred labels on unseen data; however, we found and demonstrated that training with more samples per label may result in greater success during inference.
Recommended Citation
Hinkel, Destin, "Classifying Supersonic Frequencies for Active Acoustic Side-Channel Exploitation" (2024). Theses and Dissertations. 202.
https://jagworks.southalabama.edu/theses_diss/202